There are tantalizing phenomena characterized by highly-structured collective behavior that naturally emerges over time from the interactions of more fundamental units or sub-systems, without requiring centralized control or coordination imposed from the external. It is fascinating, since new intervening mechanisms or laws spontaneously appear at scales that are not governed by the equations of motion specified at the lowest scales (see more, later). From ant colonies to flocking birds, from financial crises to hurricanes, the overall effect is summarized in the famous “the whole is larger than the sum of its parts”.
What is emergence?
That’s really a great question. I usually draft a first answer by citing my colleague Peter Dodds, who provided me years ago with a beautiful short poem to empirically describe what emergence is:
There’s no love in a carbon atom, No hurricane in a water molecule, No financial collapse in a dollar bill — P. Dodds in Complexity Explained
Another interesting one is from Murray Gell-Mann:
You don’t need something more to get something more. That’s what emergence means. — M. Gell-Mann
While, strictly speaking, there is no formal theory of emergence, it has evolved significantly from its philosophical roots in Aristotle’s notions of material substances, where complex properties such as human mental capabilities are seen as arising from, yet distinct from, simpler physical elements.
The scientific revolution shifted the Aristotelian focus towards a reductionist paradigm, through Cartesian dualism, imposing a strict separation between the material body and the immaterial mind, while advocating a mechanistic understanding of physical phenomena.
In the 19th century, British emergentists like John Stuart Mill ("Of the Composition of Causes" Chapter VI of System of Logic (1859)) and C.D. Broad (“The Mind and Its Place in Nature”) revitalized the concept by arguing that complex systems display properties not predictable from their individual components alone. This mindset was countering the reductionist trends prevailing at their time, suggesting that these properties are supervenient yet autonomous. The more recent discussions about the topic, especially within the scientific community, often focus on the ontological status of these emergent properties—whether they are fundamentally new entities or can be fully explained through underlying physical laws. The stakes are high in fields like physics, biology, and cognitive science: understanding the nature of complex systems’ behaviors and properties challenges the more simplistic reductionist explanations, supporting the idea of a less controllable and more dynamic natural world.
In 1972, in his famous paper "More is Different" Phil Anderson argued that complex systems exhibit properties and behaviors that are not merely an aggregate of their parts, suggesting that the reductionist approach in physics is insufficient for understanding emergent phenomena at higher levels of complexity.
Psychology is not applied biology, nor is biology applied chemistry. — Phil Anderson
In 2000, Laughlin et al proposed “the middle way”:
Mesoscopic organization in soft, hard, and biological matter is examined in the context of our present understanding of the principles responsible for emergent organized behavior (crystallinity, ferromagnetism, superconductivity, etc.) at long wavelengths in very large aggregations of particles. Particular attention is paid to the possibility that as-yet-undiscovered organizing principles might be at work at the mesoscopic scale, intermediate between atomic and macroscopic dimensions, and the implications of their discovery for biology and the physical sciences. The search for the existence and universality of such rules, the proof or disproof of organizing principles appropriate to the mesoscopic domain, is called the middle way.
There are entire books dedicated to emergence, the difference between weak and strong emergence, and so on. It would be impossible to summarize all those efforts.
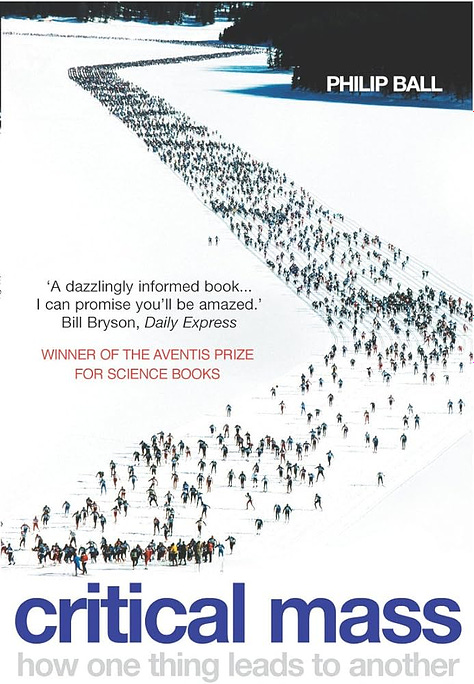
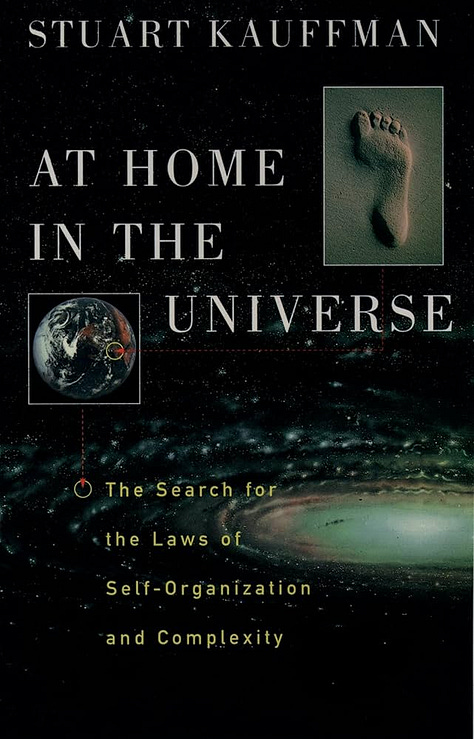
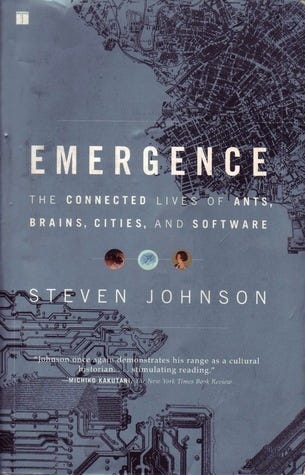
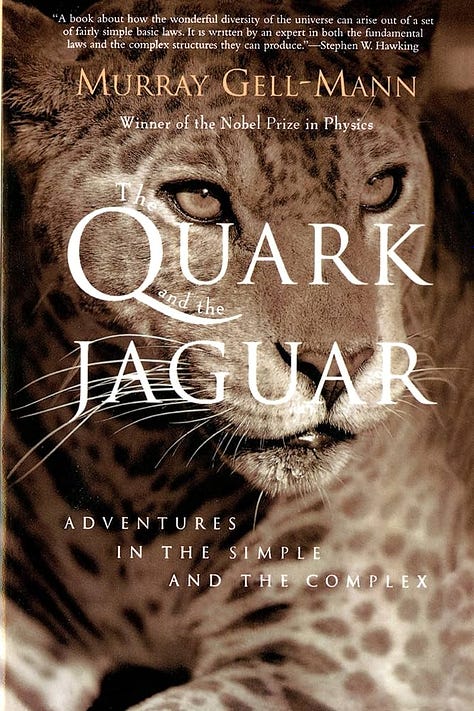
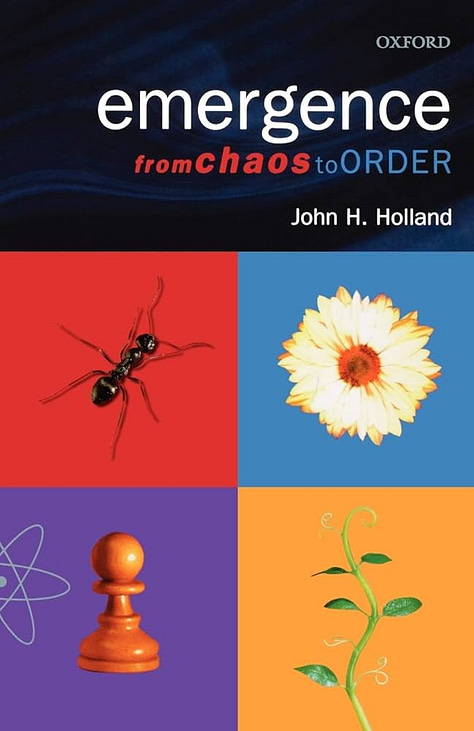
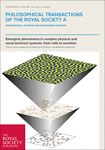
Two years ago, we have tried to gather together several experts from different disciplines to tackle together the challenge of characterizing emergent phenomena, from cells to societies. You can read our introductory paper, where we make an effort to review emergent phenomena in quantum physical systems, in classical physical/non-living systems, in living systems and in social systems.
d oijw ao o fyrg bafjdsdpw dweoda wdhao jrfgb sag wdgy d ias dsih sig qqpdjwe fjrfb dvvs. — O. Artime & M. De Domenico
Building on the fundamental works by David Chalmers and Mark Bedau, we have sketched a simplified, yet starting, formal approach to characterize emergence.
Using the above terminology, we can define a taxonomy for a variety of phenomena:
Non-emergent: one has knowledge of LLMR and IC allows us to deduce expected HLP;
Weakly emergent: one has knowledge of LLMR and IC allows us to deduce unexpected HLP through computation (e.g. simulations);
Strongly emergent: knowledge of LLMR and IC does not allow us to deduce HLP even in principle.
Here the point is not to debate weak vs strong emergence, but about the necessity to find a formal language to discuss in a constructive and operational way.
A computational approach to hierarchical emergence
In a recent pre-print, Fernando Rosas and collaborators contributed to the ongoing efforts by analyzing emergence from the perspective of how software works. This choice allows them to capitalize on a formal description of how macroscopic processes can express self-contained informational, interventional, and computational properties.
I have asked Fernando some questions to help me to better grasp the details of their proposal.
What’s the most fundamental advance brought by your paper with respect to previous attempts?
I see the field right now proceeding to formalise various “perspectives” of what emergence is. It is a bit like what happen with complexity: first we thought we needed the “right metric” of complexity, to then realise complexity means many different things, and proceed to formalise each of them. In this sense, this work belongs to a group of efforts trying to use information-theoretic tools to characterise emergence in time series.
See also:
→ Quantifying causal emergence shows that macro can beat micro
→Reconciling emergences: An information-theoretic approach to identify causal emergence in multivariate data
→Dynamical independence: Discovering emergent macroscopic processes in complex dynamical systems
A key difference between this paper and others is an effort to go beyond scalar metrics, and characterise emergence via more machinery. The approach was to focus on what “closure” of a given scale in time series data could mean. We identified three faces of “closeness”:
Information closeness: optimal predictions can be build from macro data alone,
Causal closure: every intervention that can make a difference for the macro can be done at the macro level,
Computational closure: the computations that characterise the macro can be identified by coarse-graining of the computations that characterise the micro.
The key theoretical contribution is to formalise each of these ideas and clarify the links relating them. I’m particularly excited about the bridges established between signal processing (prediction), causal discovery (interventions), and theoretical computer science (computations via automata models).
How do you think that coarse-graining can deal with interactions that happen between two distinct levels of description? Is there a limitation here of what can be captured by the framework?
Indeed. This approach captures a very specific type of emergence associated with closeness — which could be thought of as self-sufficiency. By doing this, this approach is not well suited to study inter-scale interactions, e.g. downward causation or similar phenomena. For that one has to use other approaches (see here).
By the way, it is not at all clear at this point if all these different approaches can be unified in a single one or not. Clarifying that will be important future work.
Why is important to have informational closure? What does it add with respect to the traditional prescription of statistical mechanics that you can neglect microscopic details when focusing on macroscopic ones?
I’d agree that information closure is the least exciting type of closure of the three. However, by linking information closure with causal and computational closure one can relate such stat mech notions with ideas about intervention and computation in a rigorous way. So, for example, one could not only say that one can neglect microscopic details, but can directly see how the computations are simplified by doing this. By the way, I believe there is lot of work to build these bridges more strongly, this preprint is just a first step in that direction.
Another cool feature of the proposed approach is that the various levels can be incomparable: i.e. one may not have a totally order sequence of levels but a partially ordered lattice. I believe having multiple non-comparable coarse-grainings is particularly interesting for biology and AI, but fully exploiting this is also future work.
The work is based on the concept of “epsilon-machines”, introduced by James Crutchfield as a conceptual framework to understand and analyze patterns and structures in sequences of data. Understanding and identifying the emergence of complexity in natural phenomena involves a subjective yet critical scientific approach heavily relying on how observers build models to interpret data from their environment, influenced by their computational tools (essentially, the data they can gather, the memory they have for storage, and the time they can devote to analysis). What an observer might consider ordered, random, or complex directly ties to these computational resources and their organization. The effectiveness of identifying patterns largely depends on the computational model used by the observer, which in turn can significantly impact the observer's ability to discern regularity in the data.
For instance, imagine you’re trying to predict the weather based on past weather patterns: an epsilon-machine breaks down the complex data (the weather records) into a series of states based on what's happened before. Each state in the machine represents a specific pattern in the data, and the transitions between these states (like moving from a rainy pattern to a sunny pattern) are determined by the rules the epsilon-machine creates from the data. The goal is to use the least complex set of rules and states that can accurately predict what comes next in the data (a goal that makes it compatible with the goal of the minimum description length principle proposed by Jorma Rissanen in 1978 [if you are curious, I recommend also to take a look at stochastic complexity], while keeping some relevant differences). This approach is especially useful in studying systems where patterns repeat or have some regularity, since it can help to uncover the underlying processes governing physical, biological or social complex systems.
This ambitious task cannot be simply achieved by statistical mechanics, according to Cosma Shalizi and James Crutchfield, and requires epsilon-machines as a starting point for a complementary analytical framework known as computational mechanics.
Said that, we are now ready for my next question.
Why is it necessary to consider (or: what’s the main advantage in considering) epsilon-machines at each scale?
Using epsilon-machines provide a dual representation to the data, so that the lattice of causal or informationally closed coarse-grainings has an image in epsilon-machine space that corresponds to the lattice of computationally close automata. This is useful, as typically the mapping from the lattice in “real space” to “theory space” is not 1-1, so that the latter is usually simpler. And the claim is that the shape of the second lattice is the one that gives the clearest insight about the multi-level computational structure of the system.
From Crutchfield’s work we know that epsilon-machines simplify complex data by providing a streamlined computational model that makes it easier to understand how different parts of a system interact and evolve. In this new paper, if I have understood correctly, the authors use coarse-graining to further simplify complex data into a workable structure in the space of models (the “theory space” described by epsilon-machines), being reassured that this choice is the one that provides the most parsimonious (or kind of) description of the multi-level organization of the system.
[ndr: I’d love to test this claim in terms of description length, for instance]
Finally, I was a bit confused by the example related to networks, which has been further explained in a recent piece by Philip Ball:
One [model] is a version of a random walk, where some agent wanders around haphazardly in a network that could represent, for example, the streets of a city. A city often exhibits a hierarchy of scales, with densely connected streets within neighborhoods and much more sparsely connected streets between neighborhoods. The researchers find that […] the probability of the wanderer starting in neighborhood A and ending up in neighborhood B — the macroscale behavior — remains the same regardless of which streets within A or B the walker randomly traverses.
For the simplest random walker dynamics, where the transitions rules are only local, the steady-state probability of reaching a destination (B) does not depend on the network topology and on the origin (A), since it depends only on the degree of the destination. However, this might not be the case for a variety of other random walks and more realistic dynamics. Can you better explain what’s the main message here?
This is a very good point. Our preprint is not trying to say anything general about random walks on networks, but instead using a very specific network to illustrate how computational closure works. So, the idea is that while one would tend to think of a random walk as a local process, for this particular case the computation of the next step can be decomposed into three nested tasks:
select the size of the next community,
select the identity of the community within the ones of the same size,
select the node within the selected community.
And one can prove that, thanks to the highly symmetric network structure, the computational process of each scale is close with respect to the ones below. This means that one could simulate the dynamics at any of those three levels perfectly while completely disregarding the levels below.
This makes sense as a very specific example. Nevertheless, it might be a totally different story for more complex random walks, so I will wait for more refined versions of this example, which is very important for network scientists.
Take home messages
Emergence describes how complex behaviors and properties can arise from the interactions of simple(r) units. This concept, often summarized in “the whole is greater than the sum of its parts” transcends the boundaries of disciplines, applying to physical, natural and social systems.
Understanding emergence challenges reductionist views and pushes the boundaries of how we conceptualize the outcome of interactions within systems (ecosystems, societies, economies).
The newly proposed framework, building on computational mechanics, offers a method to distinguish levels of macroscopic behavior in certain complex systems. However, its scope is (currently) limited to systems exhibiting self-sufficiency, and it might be really hard to apply the framework to empirical data of large systems. Future extensions could enhance its applicability, although it is still not clear if it can be unified, or not, with existing approaches that directly address inter-scale interactions.
Further reading: A very recent review → On principles of emergent organization
I always felt emergence was the direct consequence of interactions between components. Isolated nodes don't create any emergence; connected ones do. We are increasingly connected. I'm tempted to say interaction and emergence imply each other. No emergence without interaction, no interaction without emergence, at least in practice.
terrific post, really inspiring