AI, Cybernetics, and Complexity: unpacking the 2024 Nobel Prizes
Have the 2024 Nobel Prizes embraced complexity again after 2021?
Is #ComplexityThoughts intriguing to you? Follow us and share your thoughts! Your feedback is invaluable for this free service, and it helps shape the frequency and quality of our newsletter. Thank you for being part of our journey!
NEWS: Check out 'Our Podcast' for short discussions on our posts, now available on Spotify!
Several prominent complexity scientists have won Nobel Prizes, though not specifically for their contributions to complexity science. Murray Gell-Mann, awarded the 1969 Nobel Prize in Physics, made significant advances in complex adaptive systems. Ilya Prigogine, who won the 1977 Nobel Prize in Chemistry for his work on dissipative structures, greatly influenced non-equilibrium thermodynamics. That same year, Philip Anderson received the Physics Prize for his discoveries in condensed matter physics, yet he was also a major figure in understanding emergent phenomena. Herbert A. Simon, a founder of artificial intelligence and decision theory, won the 1978 Nobel Memorial Prize in Economic Sciences for his work on organizational decision-making. Robert B. Laughlin, awarded the 1998 Nobel Prize in Physics, was celebrated for his work on quantum fluidity, though his ideas also extended into emergent properties in complex systems. Lastly, Michael Levitt, awarded the 2013 Chemistry Nobel for computational models of biological molecules, made key contributions to understanding multiscale molecular complexity.
But I will remember 2021 as the year when complexity science was finally recognized as a Nobel Prize-worthy field within physics:
The Royal Swedish Academy of Sciences has decided to award the Nobel Prize in Physics 2021 “for groundbreaking contributions to our understanding of complex physical systems” with one half jointly to Syukuro Manabe (Princeton University, USA) and Klaus Hasselmann (Max Planck Institute for Meteorology, Hamburg, Germany) “for the physical modelling of Earth’s climate, quantifying variability and reliably predicting global warming” and the other half to Giorgio Parisi (Sapienza University of Rome, Italy) “for the discovery of the interplay of disorder and fluctuations in physical systems from atomic to planetary scales”.
The Nobel committee’s 2024 decision to award their prizes in Medicine, Physics, and Chemistry to what seem like AI-related discoveries has sparked significant discussion, crossing traditional academic boundaries (which, after all, are human-made, not natural).
However, focusing solely on the AI aspect of these discoveries would be an oversight, as they are deeply rooted in complex systems and address fundamental questions that have been explored since at least the 1940s.
In a previous post I have quickly covered some technicalities about why the Nobel prize in Physics is, you know, Physics:
In this post, I explore with two colleagues, and good friends, what these prizes mean for Complexity Science and the perspective for the next future.
“Reality has a cybernetic bias. It does not fit in departmental boundaries.” — Luis M. Rocha
Background
The 2024 Nobel Prize in Chemistry recognizes breakthroughs in protein design and structure prediction, for innovations that are transforming biotechnology.
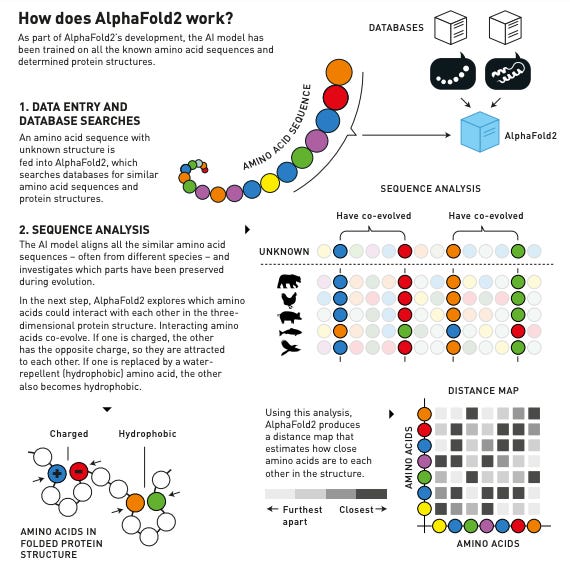
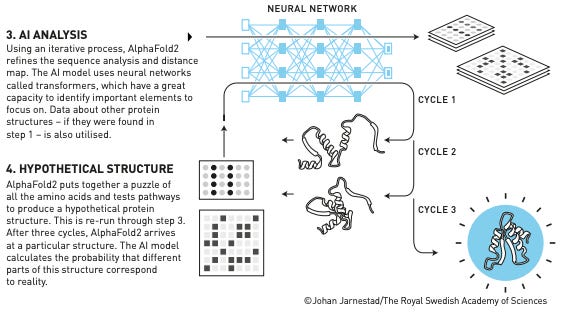
In Medicine, the award goes to the discovery of microRNAs, which revolutionized our understanding of gene regulation and how cell types differ despite identical genetic material.
The Nobel Prize in Physics honors foundational contributions to machine learning, specifically the development of neural networks and associative memory, which paved the way for modern artificial intelligence.
An interview with Prof. Ricard Solé and Prof. Luis M. Rocha
“Indeed, we live in a renaissance [of cybernetics].” — Ricard Solé
Do you think that we are a modern renaissance of cybernetics, as the boundaries between biological, computational, and physical systems blur?
Luis M. Rocha: Great question. I do believe we have essentially reached the world that Norbert Wiener articulated, especially on his follow-up to Cybernetics, “the human use of human beings.” How much people---including scientists---are aware of it, is a different question. So, yes, the key concepts from cybernetics such as computation, information, control, networks, feedback, automation, functional equivalence of organization, and complexity are a central part of explanation in almost any discipline today.
The success of cybernetics was quite stealthy; rather than winning via the establishment of departments in universities, its general-purpose concepts and discoveries became ubiquitous. Along with them comes interdisciplinarity. The need to use vast combinatorial searches to tackle complex systems (as first pointed out by Warren Weaver in 1948) made computers and distributed machine learning key in all disciplines. This creates what George Klir called a two-dimensional science, where the disciplines of systems science (another name for cybernetics) are in effect orthogonal to the traditional disciplines that are organized by their empirical scales of observation (chemistry, biology, society, mind). This orthogonality, or “general-purposiveness”, facilitates cybernetics concepts jumping from field to field, sort of hitchhiking along computational approaches.
Of course, if cybernetics concepts were not useful for explainability or predictability, they would not take hold. This reminds me of the spoof letter (Ephrussi, et al (1953). Nature 171, 701) that James Watson and others (wrote in 1952 but) published just 7 weeks prior to the famous Watson and Crick 1953 paper. In this letter they mocked the concepts of information and cybernetics. But immediately after the physical reality of the DNA molecule forced Watson and most of biology to eat their words, and use the terms “information”, “code”, “control” unironically. To paraphrase and mutate Stephen Colbert, reality has a cybernetic bias. It does not fit in departmental boundaries.
Ricard Solé: Although not much appreciated sometimes, cybernetics was the first attempt to develop a theory of complex systems. In a way, it was the first Golden Age of complexity. The understanding of neural systems was rapidly improving, and Norbert Wiener unified dynamical systems with the nature of behaviour and agency. At the same time, the view of organismal stability via feedback became a general principle for control and reliability for living and non-living machines. In parallel, the theory of computation by Turing, along with the rise of computers and the connections made by von Neumann between computers and brains (on the shoulders of Warren McCulloch and Walter Pitts) depicted the possibility for a formal understanding of the brain. Indeed, we live in a renaissance.
Considering the recognition of artificial neural networks and protein folding, where do you see the limits of our current ability to model and predict complex systems?
Luis M. Rocha: The ultimate limits are of course matter and energy. Modern AI is notoriously energetically costly. At least since Hans Bremermann’s work in the 1960s, we are aware that a computer with the mass of the Earth and its longevity cannot exhaustively search all the combinations of relatively mundane problems. Therefore, all approaches to complex systems need to simplify problems and accept uncertainty as a trade off.
But perhaps a more serious problem are complex systems whose future cannot be fully predicted from past data, or in sufficient time will deviate from any inductive model you can build from past data. Robert Rosen and Howard Pattee reserve the term complex system only for those systems. Nassim Nicholas Taleb (via Karl Popper) says the same thing by speaking about “black swans” that are arguably responsible for the major changes with greater impact in society. For instance, it is hard to imagine that an inductive machine (like artificial neural networks), given all past data about viruses and every relevant layer of nature and society, could predict what specific virus will cause the next pandemic, much less its precise zoonotic transfer and spread events. Both because a machine necessary to process all that information for precise prediction is likely impossible, and the emergence of new variants in biology might very well be open-ended, such that the never before observed arises.
This inductive limit leads some of us to think that to tackle complex systems, we need to use the power of inductive machines not to predict the entire black box, but to accurately estimate parameters of simpler, higher level causal models that not only provide explanation, but allow us to consider unobserved scenarios. The work of Alex Vespignani on epidemic forecasting comes to mind for that explainable and actionable approach.
By the way, and being more speculative, language itself may be one such strategy, whereby mixing up words that do have an inductive correspondence, allows us to consider words for concepts that were never unobserved---think unicorns. So, I tend to think that large language models may actually be on to something very interesting when, in addition to the ability to recombine previously observed language, an effective selective mechanism (perhaps with embodied repercussions) is provided.
Thinking about the foundational mechanisms discovered by the awardees in Medicine, Physics and Chemistry, are we closer than ever to complexity as new frontier of science?
Ricard Solé: The trend is inevitable. Some disciplines, like neuroscience and ecology, have traditionally been systems-level sciences, where considering multiple scales and global properties emerging from simple rules was common. The Human Genome Project and, in general, the flood of data that started to pour down in the early 2000s revealed something that was ignored by most experts in those areas: we cannot explain much by looking at the molecular detail. This is why systems biology is becoming a strong part of the life sciences.
In the light of the awards for those foundational mechanisms, are we scratching the surface of what it means to understand emergent systems? From life to cognition, to be precise.
Ricard Solé: I think so. Emergence is a central concept within complexity sciences and, unfortunately, is still unknown to many. There is a long tradition in physics concerning the nature of phase transitions and building theories that explain macroscopic properties from very simple models. We know that interactions can be much more important than the nature and details of the units. That is a huge achievement and is percolating across disciplines.
Does the rise of interdisciplinary Nobel Prizes signal that the future of groundbreaking science lies in merging traditionally separate fields? Or do you think that the Nobel committee jumped into the AI-hype bandwagon?
Luis M. Rocha: I think both are likely true.
Ricard Solé: Hopfield's work inspired thousands of papers and books in physics. Physicists immediately understood that an extension of spin glasses could have enormous explanatory power in relation to cognitive systems. On the other hand, Hinton worked with models that, again, are easily connected to physics (and inspired by the multilayer picture revealed by neuroscience), which paved the way for a revolution in artificial neural nets and computational neuroscience. Not surprisingly, many physicists also jumped into that. So, this is a deserved recognition of the success of the power of interdisciplinarity.
Do you think the focus on systems-level understanding (whether in AI, biology, chemistry or physics) signals a fundamental shift in how we approach scientific problems?
Luis M. Rocha: From my answer above, I think this shift has not been sudden, but rather a stealthy but steady revolution started with the cyberneticians.
Is reductionism fading in favor of complexity science, where interactions and emergent behaviors hold the key to solving some of the biggest challenges?
Ricard Solé: The analytical view, grounded in the decomposition of its parts, has worked well and has been instrumental in developing biomedical research. This will never be replaced when dealing with drug design, understanding cancer, or immune systems (to mention just three examples). We need research that looks into that, and big data and machine learning open a fascinating future. This is, however, part of a story where multiple scales of complexity exist and cannot be explained by looking only at the small scales. What seems clear is that a whole repertoire of problems, from life origins to higher brain functions, complex diseases, or climate change, will only be solved using the systems-level view.
Luis M. Rocha: Complex systems science should have a balanced and healthy mix of reductionism and “emergentism”, or complementarity (per Goguen and Varela, or Howard Pattee). The question should be what the appropriate level of organization is to minimize model complexity while maximizing predictability and explainability. Sometimes reducing provides the best explanation, sometimes considering higher-level organization (interactions, information, etc) is best.
To exemplify let me go back to the genetic code example. Often, we hear people say that code and information is just a metaphor to explain genes. First, all models are metaphors. So, what is important is choosing the simplest model that grants greater predictability. Clearly, one can reduce DNA to its molecular constituents and even to its quantum physics, but the most parsimonious way to predict the amino acid sequence that the ribosome produces is the information stored in sequences of 4 nucleotide letters (while ignoring their chemical and quantum basis) . We could choose not to use the information level and rather model the process at lower levels, but that would greatly increase the complexity of the model without a gain in explainability or predictability. This means that for this phenomenon, the correct level is of DNA as information---thus Watson’s change of heart mentioned above.
The same principle applies in other phenomena. For instance, in psychology, much evidence exists now that language therapy (e.g. Cognitive Behavioral Therapy) is often more effective than pharmacology. This suggests that the appropriate, at least most effective, level for mechanistic explanation of depression might be linguistic, rather than neurological or molecular.
I thank Ricard and Luis for this nice discussion.
The future of complex systems is now, and data and computing power will not be enough to deal with them.
“it is hard to imagine that an inductive machine, given all past data about viruses and every relevant layer of nature and society, could predict what specific virus will cause the next pandemic” — Luis M. Rocha
“What seems clear is that a whole repertoire of problems, from life origins to higher brain functions, complex diseases, or climate change, will only be solved using the systems-level view. ” — Ricard Solé