Did you know that the number of UFO sightings in Missouri nearly perfectly correlates with the consumption of petroleum in Kuwait? It can’t be a coincidence and the most plausible explanation is that UFO fuel their starships in Kuwait before taking off. We all know that Kuwait is plenty of petroleum, after all.
But we can do better and ask an AI about other likely explanations (see the source here):
Perhaps the UFOs were really just giant, energy-hungry space snails on a secret mission to fuel up on Earth's oil reserves and Missouri was their intergalactic pit stop, leading to an inadvertent spike in petroleum consumption in Kuwait as they zoomed off back into the cosmos. After all, you can't expect extraterrestrial visitors to carpool, can you?
Can you believe it that the main responsible of divorces in Maine, USA, is the consuption of margarine per capita? The correlation is perfect:
And what about the air quality in Chicago? Did you know that it increased in the last two decades at the same rate at which hydropower energy was generated in Greenland? Again, it can’t be a coincidence: maybe Greenland is polluting less and since it’s “close” to Chicago, the city is benefitting from the green policies of that cold country.
But wait… it seems that the air quality if Chicago correlates pretty well also with the popularity of the “red pill/blue pill” meme…
and with the stock price of Walt Disney!?
But also the correlation with the annual revenue of the Lego group is astonishing:
What’s going on here? Tyler Vigen, who discovered those amazing correlations explain them here:
Data dredging: I have 25,237 variables in my database. I compare all these variables against each other to find ones that randomly match up. That's 636,906,169 correlation calculations! This is called “data dredging.” Instead of starting with a hypothesis and testing it, I instead abused the data to see what correlations shake out. It’s a dangerous way to go about analysis, because any sufficiently large dataset will yield strong correlations completely at random.
Lack of causal connection: There is probably no direct connection between these variables, despite what the AI says above. This is exacerbated by the fact that I used "Years" as the base variable. Lots of things happen in a year that are not related to each other! Most studies would use something like "one person" in stead of "one year" to be the "thing" studied.
Observations not independent: For many variables, sequential years are not independent of each other. If a population of people is continuously doing something every day, there is no reason to think they would suddenly change how they are doing that thing on January 1. A simple p-value calculation does not take this into account, so mathematically it appears less probable than it really is.
Outlandish outliers: There are "outliers" in this data. They stand out on the scatterplot above: notice the dots that are far away from any other dots. I intentionally mishandeled outliers, which makes the correlation look extra strong.
At this point you might smile and think that most likely you have never had a similar problem in your research, since you use data that measured in your own experiments and “there must be a causal relationship” in so controlled environments, right? Well, let’s ask Mrs. Statistics what she thinks about it.
In 2010 a group of scientists reported an “incredible discovery”:
[…] we completed an fMRI scanning session with a post-mortem Atlantic Salmon as the subject. The salmon was shown the same social perspective taking task that was later administered to a group of human subjects.
Imagine the surprise to find a signal when scanning the brain of a dead salmon (Salmo salar, approximately 18 inches long, weighed 3.8 lbs), and in fact the study has later been awarded the IgNobel prize in neuroscience.
However, the study was interesting for a good reason: it was devoted to show how current practices, neglecting specific basic aspects of statistics (like correcting for multiple comparisons), can lead to wrong results.
In fact, similar studies are based on t-contrast for regions with BOLD signal that significantly change during the task with respect to rest, and then apply some (usually arbitrarily chosen) threshold. Given the large number of tests being performed (there are more than 100,000 voxels to test) the probability to have spurious signals (i.e., false positives) is high enough. As the authors have shown, it is enough to use statistics controlling for the familywise error rate (FWER) and false discovery rate (FDR) to dramatically reduce the probability of finding a signal where there is no signal, even at relaxed statistical thresholds.
The dead salmon test is not the only example. As scientists we look for patterns: and, unfortunately, if you look for a pattern you will almost probably find it. Take, for instance, the image of the moon that after being zoomed out revealed the presence of a face, or the face captured on the surface of Mars by the Viking 1 mission in 1976:
It’s pretty clear, it’s there: can we interpret it and understand what’s going on?
Let’s move to cosmology, where a lot of smart scientists deal every day with tons of data, mostly images, and they have to look for patterns deviating from what one would expect by chance. This is the routine for data such as the cosmic microwave background (CMB) radiation. They have to, since the presence of more (or less) signal where you don’t expect it can be a strong indicator of phenomena of cosmological interest.
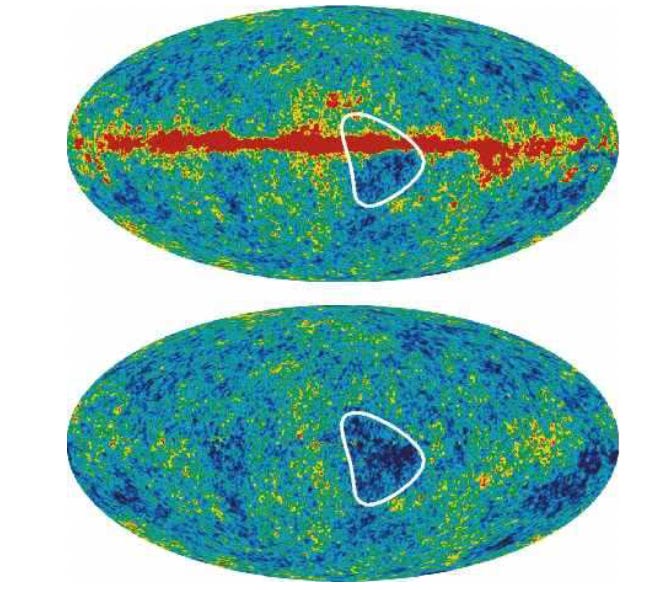
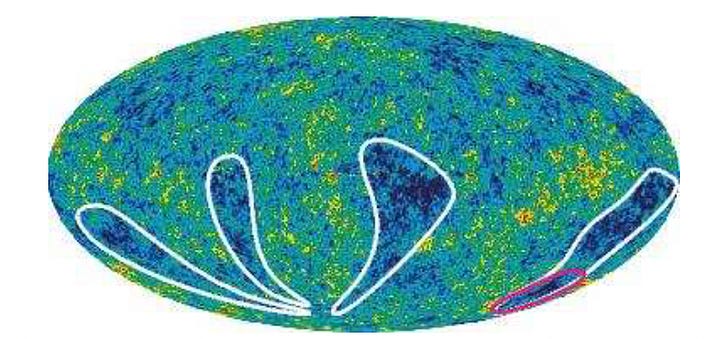
In a 2010 paper they have analyzed the latest available data and reported an intriguing discovery: they have found the letters “SH” in their CMB map. Why that’s interesting? Because they are, by coincidence, the initials of Stephen Hawking, one of the most influential scientists in cosmology.
Very cool: the CMB signature was connected to Hawking by some unknown and invisible forces of the universe. After all, if you now compare “this fact” against millions or billions of simulations coming from random or sophisticated models, you will find that this specific large-scale pattern (i.e., the letters SH) cannot be easily found. The obvious conclusion is that such a pattern must have a strong cosmological meaning: yay!
Why? You have simulated N times (with N ~ 1 trillion) your expected CMB according to your knowledge of the universe, and you could never find the pattern SH: that means that its probability to appear by chance must be smaller that 1/N. It’s a fact. This should be more than enough to claim a discovery for new cosmology!
But wait for a second. Why are you searching for the SH pattern only? Because you have observed it and, a posteriori, you are now performing a search for this pattern and are optimizing for it. In maximum likelihood estimation (the same that they use), the goal is to find the set of parameters that maximize the likelihood of the observed data. It is a very powerful approach when the model and parameters are specified before looking at the data. However, when applied a posteriori — i.e., after taking a look at the data — it can lead to identifying patterns that are not actually statistically significant, but rather coincidences or artifacts of the specific dataset being analyzed. After their paper, someone started a contest about finding shapes of any form in the CMB map, such as animals, unicorns, etc: due to the vast number of potential patterns and the complex nature of the data, it is almost granted to find something that appears unusual or “significant” to a likelihood test.
What do we learn from this? That proceeding in science using a similar approach can be considered identical to seeing patterns in the coffee grounds or in the clouds, or just as reading the hand for fortune-telling.
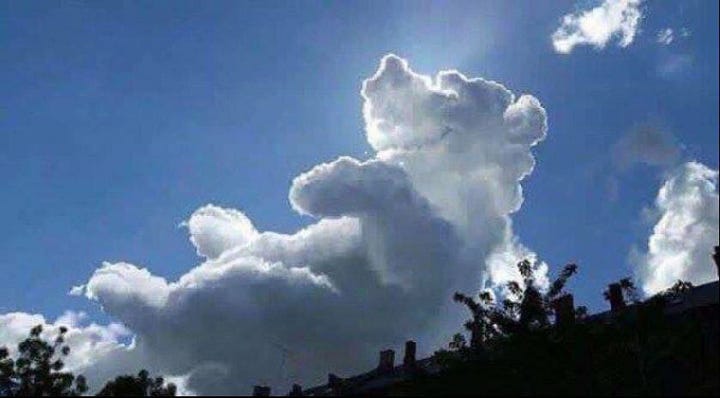
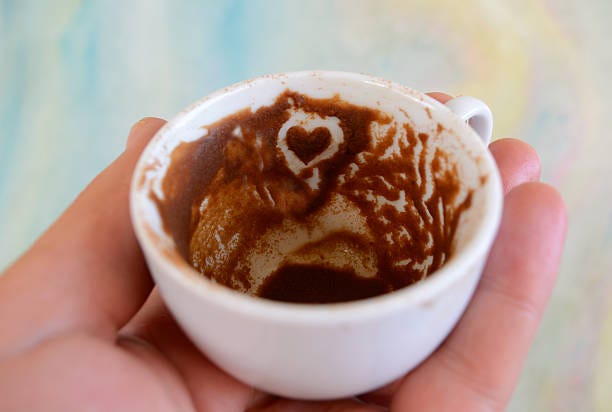
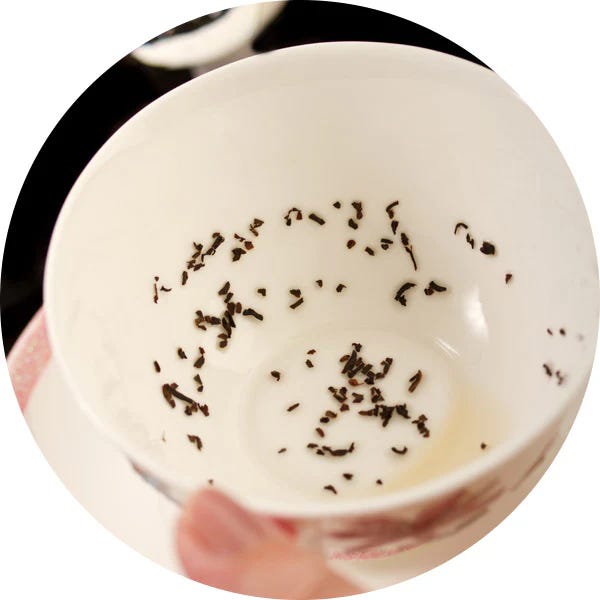
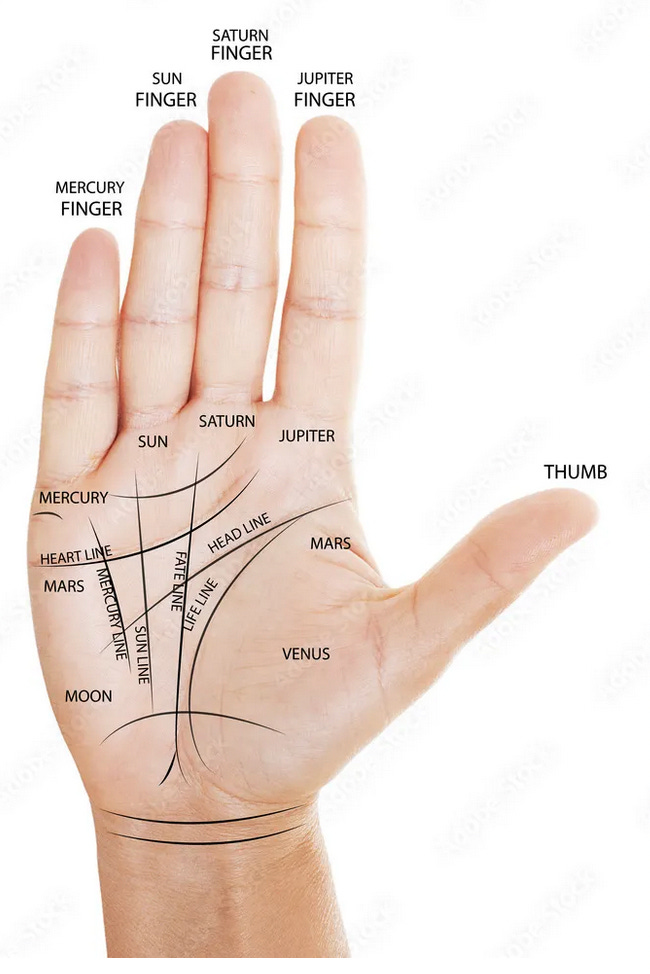
The key point is the importance of specifying hypotheses prior to data analysis to avoid the biases (such as confirmation bias) that can come from a posteriori analysis.
That’s fine: what if we look for any possible pattern as an alternative? Without being biased by the SH pattern, we might look for all the letters of the English alphabet and, why not, for the letters of the Chinese alphabet, in groups of 2, 3, 4, …
Then, if we compare our pattern (a group of letters from some alphabet) with the result of our N simulations, we can still assign a probability and maybe we are lucky enough to find a significant pattern.
As you can imagine, also this approach is wrong. Because even if you are not looking for a pattern observed a posteriori, you are now performing a systematic scan over a set of shapes (but it could be time series signals, network motifs, etc: patterns of any type) and to be fair you should penalize your analysis for such a gargantuan attempt.
This is well known in statistics (especially in particle physics) as the look-elsewhere effect: a statistical phenomenon occuring when you examine a large set of data while looking for patterns without a specific hypothesis in mind. As the number of comparisons or tests increases, so does the probability of finding at least one statistically significant but spurious result purely by chance. Actually, this is exactly the phenomenon that led to finding blinking voxels in the brain of a dead salmon watching pictures. The Wikipedia entry for this phenomenon shows how equidistant letter sequences "wiki" and "Pedia" were found in the King James Version of Genesis (10:7-14). Quite interesting, isn’t it?
You might smile, but unfortunately this is still a big issue in those fields where data is abundant and every week new methods/algorithms are proposed to get new insights.
Network science is not immune (see this paper). My colleague and friend Tiago Peixoto makes a great job in explaining why this is the case when we look for communities in our complex networks. Actually, if you generate an Erdos-Renyi network (i.e., a random network with no community structure at all), you can always find an ordering of nodes that will make your adjacency matrices looking nicely, highlighting the “presence of blocks” that are the usual signature of the presence of groups in the network.
What is the key point? Again, tha if you look for an a posteriori pattern in your data, you will find it. If you look for any possible pattern, you will find something in your data. The example usually brought by Tiago to make the point is that you can even find the face of Jesus Christ, if you have enough data and torture it long enough.
A way to mitigate this undesirable effects is to clearly define your hypotheses (i.e., a model) before you analyze the data: once you have a generative model in mind, you can use the Bayes theorem to quantify the strength of evidence for that model according to that data. [The pic below is taken from here]
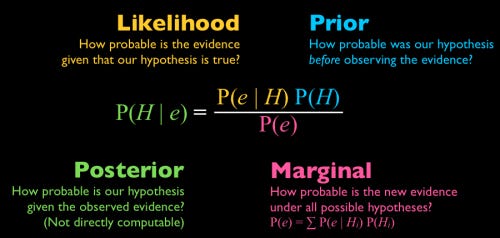
This approach is formally linked to information theory and the fact that finding regularities in the data corresponds, in fact, to find an optimal compression for it. It can be analytically shown that, under broad conditions, maximizing the Bayes posterior to fit a model or to perform model selection is equivalent to minimize the description length:
This is a powerful concept: you need to spend some bits of information to describe your data according to a given model (the first term in the right-hand side) and you need to spend some bits of information to describe the model as well (the second term in the right-hand side). Being driven by the minimum description length principle is equivalent to being driven by the Occam’s razor:
“Entia non sunt multiplicanda praeter necessitatem” — W. Ockham
Or, as it is usually described: “the simplest explanation is usually the best one”.
The problem extends well beyond network science. For instance, fields such as single-cell transcriptomics constantly needs methods to make sense of large data sets. Popular methods are based on dimensionality reduction grounded on some notion of geometry. Methods like UMAP and t-SNE are routinely used to embed large-scale data, with the goal of finding meaningful (geometric) patterns. For instance, this is a widespread approach for the analysis of single-cell data:
However, a blind application of these methods (as usual, for any method) might lead to undesired outcomes, as recently reported:
Therefore, trying to interpret the geometric patterns found by methods like UMAP is not that different from trying to find a unicorn in the clouds.
Take home message
Finding spurious patterns is easier than one can imagine. A posteriori analyses are biased by design, while systematic analyses lacking specific hypotheses should be penalized for the look-elsewhere effect. Analyzing large amount of data with a poor analytical design will inevitably lead to spurious results.
A safe approach is to follow the standard scientific method (yes, the one popularized by Galileo Galilei) to systematically observing, experimenting, and logically analyzing phenomena to develop testable hypotheses and falsifiable theories.
The objective of data analysis must be to test a meaningful hypothesis, not to find signals for free. This is not news: there are several examples, e.g. from neuroscience, advocating about this (2009, 2017, 2023) and several others can be found from other disciplines. The point is that we should make an effort to enforce good practices, even if this will inevitably lead to find less signals and, accordingly, publish less papers.
An amazing amount of insight and ideas in this. I love exploring these patterns and our human habit/need to fit this, and all the more interesting with some science behind it. Subscribing!